Unsupervised Artificial Intelligence techniques for data-driven discoveries
https://www.progetto-goforit.it/wp-content/uploads/2022/01/cer1.png
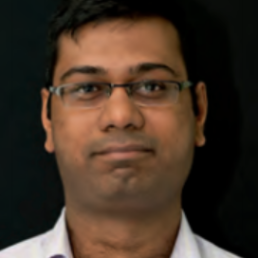
Syed Anwar Ul Hasan
Dipartimento:
Classe di Scienze, Laboratorio NEST
Abstract
In this work, entirely done at CERN, we assess the computing needs to deploy deep learning (DL) algorithms of typical size on CPUs and GPUs and compare the corresponding inference time and memory footprint to the typical requirements of an online and offline system. The question we ask in this work is very relevant for the operation of DL algorithms in the High-Level Trigger (HLT) computing farms of the ATLAS and CMS experiments during the incoming Run of the Large Hadron Collider (LHC). When using ONNX Runtime, one can keep the CPU inference time within 10 ms for as many as 64 inferences, and similarly we observe GPU inference time within 3 ms for as many as 128 inferences. This result suggests that TensorRT based compression could be an ideal choice for HLT inference with GPUs for models of our kind, size. and complexity.